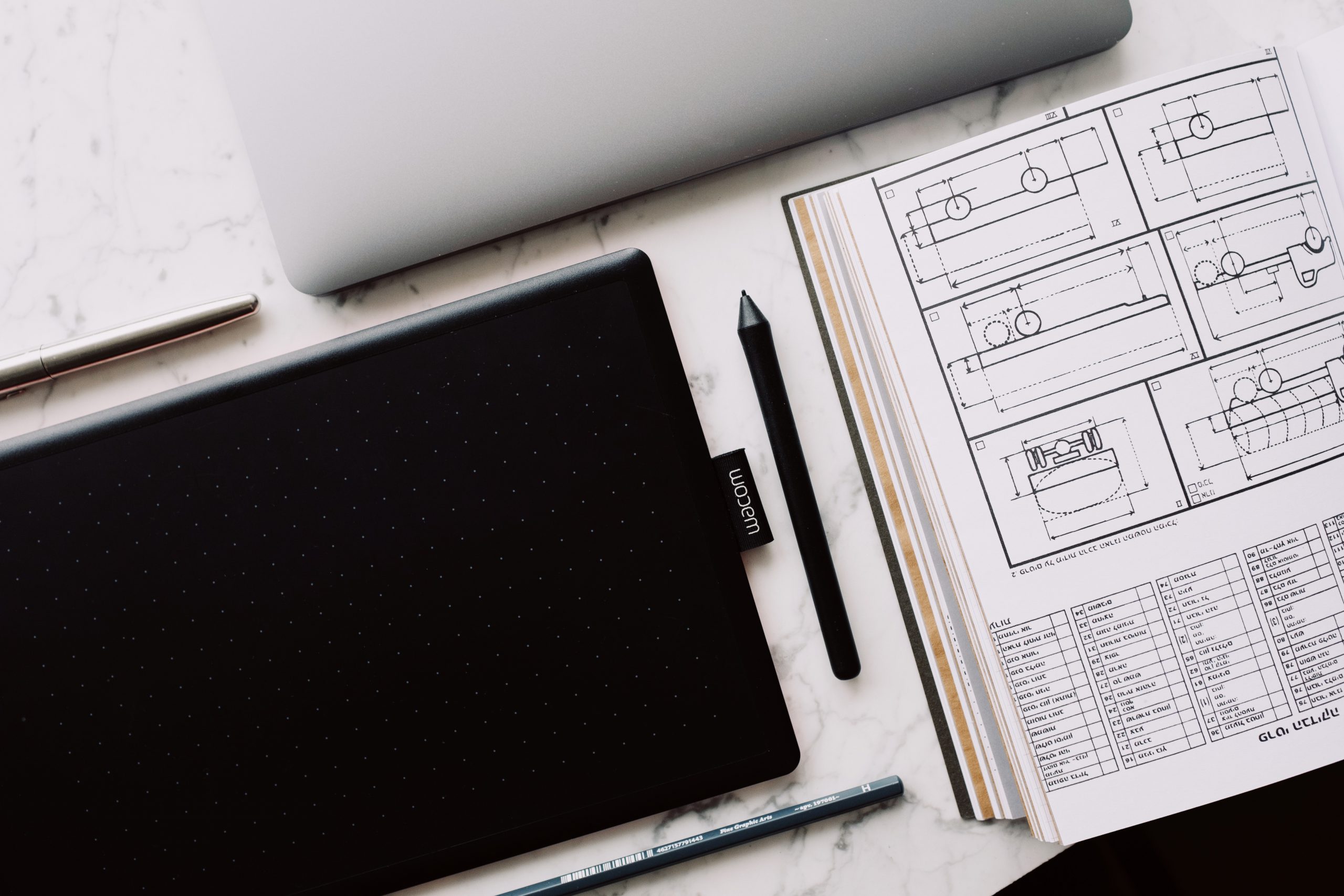
How can we measure the effects of empirical research?
Aya Mizutani
- Training Quality and Relevance
In recent years, there has been a growing trend to use randomized controlled trials (RCTs) in the development field to measure the effects of interventions. Whatever the experimental design is, it is essential to compare before and after the outcomes to see the benefit of an intervention. To measure the effect correctly, what do we need to consider in designing a pre-/post-assessment?
One of the most well-known factors that can influence the outcome is the Hawthorne effect. The Hawthorne effect was discovered in the late 1920s when researchers investigated ways to increase worker productivity. They found that, even in poor working conditions, worker productivity increased as a function of workers’ awareness of being observed; in particular, this affected their motivation and behavior to meet the researchers’ expectations. (Ohashi & Takebayashi, 2008). The Hawthorne effect is observed in RCTs as well as in the general group experiments and before/after comparisons.
Second, the effect of an intervention may be affected by dissatisfaction or lack of motivation. For example, if participants in an RCT are divided into treatment and control groups, the participants assigned to the control group may feel dissatisfied or lose motivation, resulting in lower productivity. Considering that many papers have shown that motivation contributes to productivity, this factor must be taken into account when conducting an intervention in a project.
In RCTs, we also need to consider spillover effects. Sometimes, people who are in the control group can enjoy the benefit of the intervention, for example, through information sharing or technology transfer by those who participated in the intervention. In this case, a simple comparison of the treatment group with the control group may not show the true effect of the intervention. Likewise, the effect of the intervention may not be reflected correctly because people who are assigned in the treatment group do not actually participate in the intended intervention.
However, those who are in the treatment group but did not participate in the intervention program must be included in the treatment group rather than excluded from the analysis. This is called an Intention to Treat (ITT) analysis (Gupta, 2011), which specifies that once participants are randomized, they must remain in the assigned group in the analysis regardless of dropout from or unintentional participation in the intervention. This is because participants who fall out of the intervention, or who move to the treatment group from the control group, probably have different characteristics than those who stay in the same group, and their exclusion would undermine the randomization and lead to bias.
To correctly assess the effect of an intervention, various unintended factors need to be taken into account beforehand. If the analysis is conducted without considering these factors, it may be difficult to see the actual effect.
References
- Gupta S. K. (2011). Intention-to-treat concept: A review. Perspectives in clinical research, 2(3), 109–112. https://doi.org/10.4103/2229-3485.83221
- ÖZLER B (2016) Definitions in RCTs with interference. World Bank Blogs. https://blogs.worldbank.org/impactevaluations/definitions-rcts-interference, (2021-05-10)
- 大橋昭一、竹林浩志『ホーソン実験の研究』同文館出版、2008年。